Recent advances in AI have taken the technology from a simple talking point to an essential tool across all industries. For many companies looking to optimize traditional and legacy problems, even modest productivity gains from AI could provide a market advantage.. However, some industries continue to lag behind, especially when it comes to managing their spare parts.
The hesitation might be due, in part, to the fact that spare parts workflows are built on complex supply chains and operations, as well as highly traditional processes, which make it difficult to integrate AI.
Spare parts management is inherently risky. Because of this, many companies are wary of the potential disruption to their operations that could happen from adopting what may be a fleeting trend. This reluctance has been evident over the past decade, as Industry 4.0 has grown in importance. Valtech's research supports this, showing that the manufacturing industry is cautious about adopting new technologies, fearing disrupting — and potentially compromising — established ways of doing things. In this study, they also say,
“Unlike digital-native or SaaS companies where many best practices in digital transformation have come from, traditional B2B manufacturers face a unique legacy and operational focus. Their revenue depends on physical products, with extensive operations involving production, supply chains and warehouses. This influences the organizational structure, talent pool, cultures, and well-established processes.”
How can we use AI to digitize spare parts management?
Where AI can make a real difference: Getting past the "hype"
However, it would be a mistake to compare recent advancements in AI with the "hyped" innovations of the past decade. Many of these pre-AI innovations failed to gain traction because they seemed to promise to solve all parts availability problems, but were overly complex and required significant IT support to be used effectively. One example of this has been additive manufacturing, which initially seemed to offer a solution to the spare parts problem but is actually quite difficult to scale due to post-processing requirements like sanding or the inability to scale production quickly.
Recently, AI has been on the opposite side of this spectrum. The technology has been widely adopted in almost every industry and profession by simplifying complex processes for non-experts. We could already see this happening in late 2022 when ChatGPT was first released. People could start using it immediately without having to watch instructional videos. While there are some nuances to using ChatGPT, for most people, it performs tasks as requested without requiring complicated prompt engineering.
Thanks to recent advancements with ChatGPT, AI has gone from a buzzword to opening the doors to other machine learning applications. AI can make a real difference for other Industry 4.0 technologies by simplifying their use. For example, spare parts inventory management software was oftentimes previously too complicated, had low adoption rates, and caused inaccuracies in spare parts inventory. Users were more likely to complete spare parts management tasks if the tools had AI features that made completing steps simpler and faster by utilizing better data extraction.

Innovation has historically been trapped in R&D departments
If we look back to the pre-2020 era, AI was confined to early adopters and technically savvy experts. Back then, the adoption of digital technologies was primarily limited to research and development (R&D) departments, where infrastructure, budgets, and processes were available for experimentation with innovation.
This cautious approach also limited the potential for new ideas to significantly benefit the business, particularly when it came to solving persistent problems such as spare parts management. By confining digital innovation to the R&D department as a testing ground, companies inadvertently prevented these technologies from driving widespread change and innovation throughout the organization.
In this article, we look at how companies can start effectively digitizing their spare parts management, what they should look out for in the process, and where AI can add the most value to their operations.
Change your mind — for every challenge ask yourself whether AI can help
With a number of emerging technologies now available, it's worth evaluating your current spare parts management processes to identify areas that could benefit from digitization through AI. By focusing on processes that are known to be challenging or error-prone, you can also make it much easier for your team and company to adopt the new technology as well.
For example, when you set a clear goal, you might decide to reduce duplicate part entries from 15% to 0%. This targeted approach not only clarifies the goals for digitizing the process but also provides a measurable metric for evaluating success and gives you a general sense of direction when you start looking for solutions.

An overview of where AI can help in digitization
Not sure which aspect of AI to prioritize? Start with AI's inherent strengths: data cleaning, analysis, and predictive forecasting. This set of capabilities fits seamlessly into spare parts management. Every piece of information associated with a part can be digitized and leveraged effectively through AI-driven tools to fix most spare parts management challenges today.
Data capture
At the heart of successful spare parts management is efficient data collection. AI can significantly improve this process by automating the extraction of data from various sources, such as invoices, purchase orders, and maintenance records. Through the use of optical character recognition (OCR) technology, AI algorithms can quickly scan and digitize data from unstructured formats, significantly reducing the time and effort required for manual data entry. In addition, AI-powered data capture systems can intelligently validate and cross-reference information to maintain the accuracy and completeness of spare parts inventory databases.
Advanced AI can also improve data collection and extract valuable insights from unstructured data sources such as maintenance records, sensor readouts, and service reports. By analyzing diverse data sets, AI can identify patterns, trends, and correlations that human operators might miss. This enables proactive maintenance strategies and optimized parts procurement. This holistic approach to data collection enables organizations to make data-driven decisions, improve asset reliability, and minimize downtime, ultimately increasing operational efficiency and reducing costs.
Data cleaning
Data cleaning, especially given the vast amount of data generated by modern industrial systems, is a critical yet time-consuming task in spare parts management. To automate and streamline this process and ensure data integrity and reliability, AI offers a game-changing solution. Using machine learning algorithms, AI systems can detect and correct errors, inconsistencies, and anomalies in spare parts data, improving its quality and usability. In addition, AI-driven data cleaning tools can adapt and self-improve over time. They learn from past corrections to continually improve their accuracy and effectiveness (including using external datasets to enrich uncleaned data sets).
In addition to error detection and correction, AI-driven data cleaning can also enrich spare parts data by standardizing formats, resolving duplicates, and filling in missing information. Using natural language processing (NLP) techniques, AI systems can extract valuable metadata from unstructured text fields, such as part descriptions and specifications. This further enhances the detail and richness of parts data.
Big data-based prediction modeling
In the era of big data, companies have access to large amounts of historical and real-time data that can be used for predictive modeling in spare parts management. AI techniques, such as machine and deep learning, make it possible to develop complex predictive models to anticipate equipment failures, fluctuating demand, and supply chain disruptions. By analyzing historical usage patterns, maintenance records, and environmental factors, predictive models can forecast spare parts demand with remarkable accuracy. This enables proactive inventory management and resource allocation.
AI algorithms can also continuously learn and adapt to evolving data patterns, improving predictive accuracy and reliability over time. By integrating real-time sensor data and Internet of Things (IoT) devices into predictive models, organizations can implement proactive maintenance strategies, minimize unplanned downtime, and optimize spare parts inventory levels. In addition, AI-powered predictive modeling enables scenario analysis and what-if simulations, giving decision-makers the ability to evaluate different strategies and effectively mitigate risks.
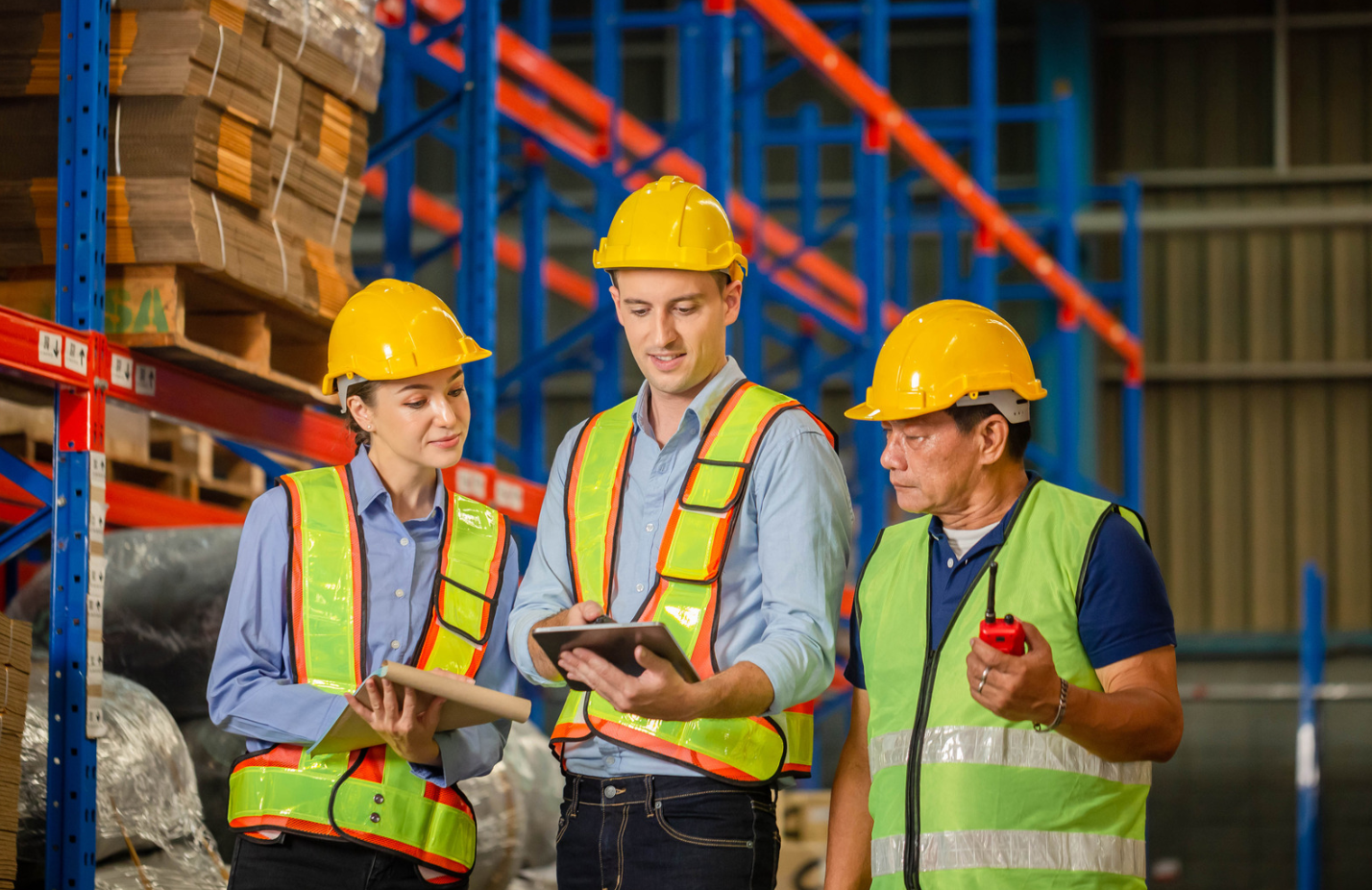
Wrapping up: Digital transformation for manufacturers in the age of AI
From tracking vast inventories across multiple locations to ensuring that obsolescence notifications are not overlooked, spare parts management has long been a significant challenge for organizations. The sheer volume and complexity of this task has made it nearly impossible for humans, or even dedicated teams, to manage effectively. This has often resulted in accepted inefficiencies and unplanned costs.
However, with the advent of AI and machine learning (ML), a clear solution is now at hand. These technologies are transforming what was once an insurmountable problem into a streamlined, efficient process by enabling the digitization and intelligent management of spare parts.
Innovative tools such as Sparrow are able to analyze, understand, and correct internal spare parts data, easing the burden of managing large inventories. Through the use of AI, companies can prevent unplanned downtime, avoid missing critical parts, and simplify procurement processes, ultimately leading to significant operational improvements.
Check out our second article in this series, which details how AI can be used to help you manage your spare parts by optimizing your inventory, decision-making, and demand forecasting.