What does it take to reduce downtime in manufacturing? The answer, increasingly, lies in the transformative role of AI in spare parts management. Ensuring the availability, stocking, and accessibility of essential spare parts for critical equipment is a core success factor for companies. It’s not just about having the right parts on hand, but also reducing operational hiccups, minimizing inventory costs, and improving maintenance teams’ efficiency.
So where does artificial intelligence, or AI, fit into this picture? AI refers to software adept at tasks that typically require human-like cognitive abilities—learning, problem-solving, and decision-making. These systems often employ technologies such as machine learning, natural language processing, and data analytics.
Efficient spare parts management is not a luxury; it's a necessity. AI offers novel solutions to long-standing challenges in the field—and as such, we’re already experiencing its impact. In this article, we’ll examine the role of AI in spare parts management and how it’s reshaping the landscape.
AI in Spare Parts Management: The Role of AI in Optimizing Inventory, Decision-Making, & Demand Forecasting
Old Methods, New Challenges: Rethinking Spare Parts Management in the Age of AI
To understand how AI is shaping the future of spare parts, we need to understand the challenges in managing spare parts inventory. One problem is high carrying costs, especially for specialized or rarely-used parts that may collect dust in a warehouse for long periods. Obsolescence is another issue; updates to machinery or new models can render existing spare parts useless overnight. And let's not forget the uncertainties that plague any supply chain—fluctuations in demand, disruptions in supply, and logistical bottlenecks create a complex puzzle that businesses must solve.
Traditionally, companies have tackled these challenges using manual data entry and spreadsheets, supported by rule-of-thumb strategies to decide what and when to stock. Such methods aren’t just outdated—they’re also error-prone. Mistakes in data entry can lead to inefficient stocking and higher costs as well as well as cause problems that ripple across an organization.
Luckily, technology—and specifically, AI—can significantly reduce the scope for such errors. While it can help streamline these processes, it’s not a one-size-fits-all solution. Nevertheless, it opens up new avenues for tackling the specific challenges of spare parts management through a data-driven approach.
Leveraging AI for Smarter Inventory Control
One of the first areas where AI makes a tangible difference is in inventory optimization.
Instead of relying solely on manual inputs and basic software, AI can assist in maintaining and updating master data. This is crucial for effective inventory planning and goes beyond just keeping tabs on numbers. Instead, it’s about organizing a complex set of data points—from cost metrics to supplier histories—to make better stocking decisions.
Moreover, AI tools are increasingly designed to access real-time data from multiple sources, providing a level of agility in responding to shifts in demand or supply chain disruptions.
Planning algorithms can then use better data to dynamically recommend optimal inventory levels, balancing the need for availability against the cost of overstocking. In addition, machine learning models can detect plant-specific usage patterns and use these to develop stocking strategies that combine better data and optimal decision-making.
Typically, this is where predictive maintenance is mentioned as a cure-all for maintenance. However, to effectively manage spare parts, you need to predict demand in the exact timespan needed to get a new part in your stock—a capability that’s currently beyond the scope of most predictive maintenance software.
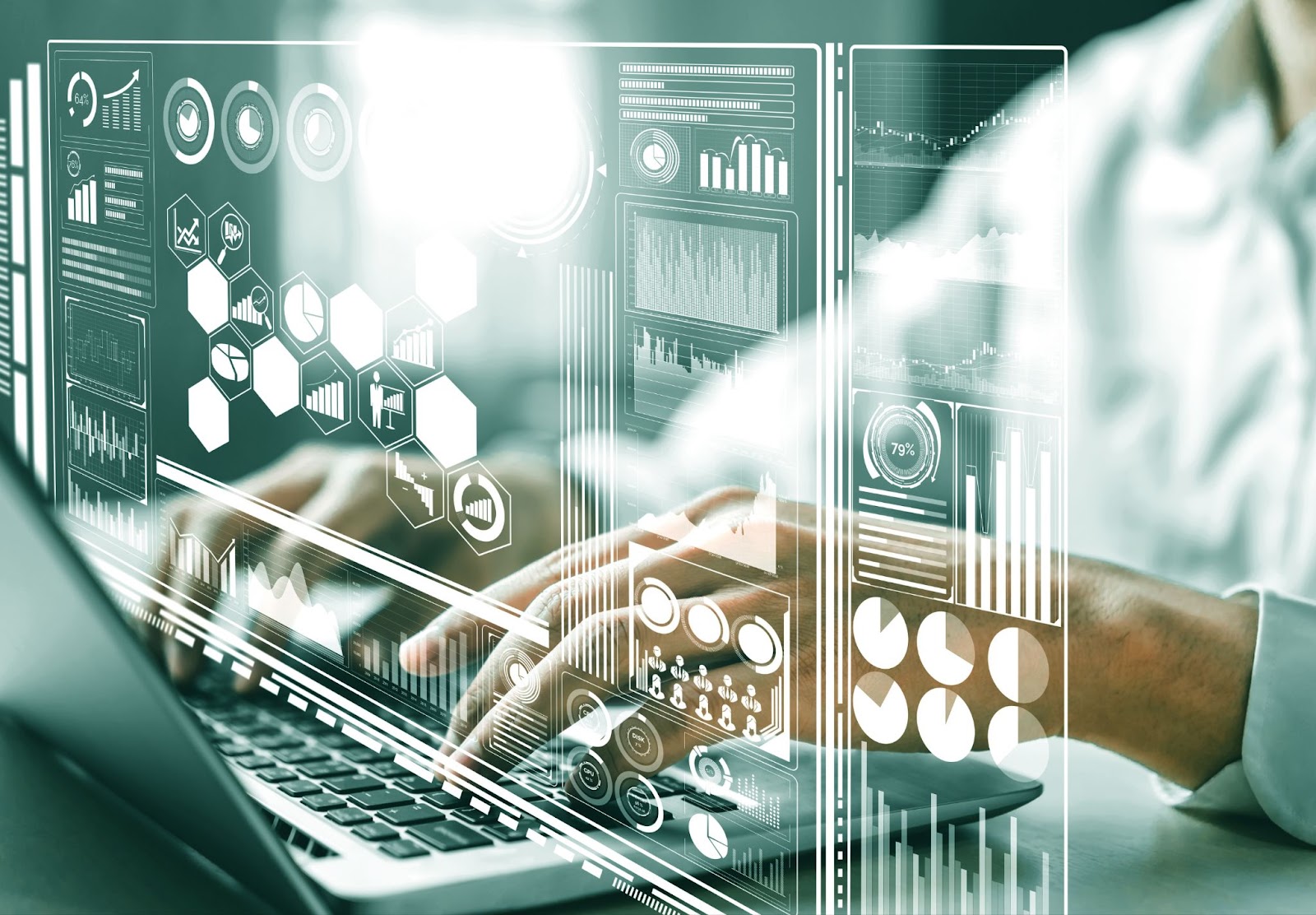
AI as a Co-Pilot for Data-Driven Decisions: Managing Spare Parts Risk
AI not only aids in managing inventory but can also serve as a valuable co-pilot in decision-making processes. One powerful example of this is demand forecasting. By sifting through historical data, AI algorithms can identify trends and seasonality that manual analysis might miss. Machine learning can go a step further to uncover recurring patterns, such as supplier delays, that can affect your supply chain. This results in forecasts that are tailored to individual part profiles, the specific machines they fit into, and even broader industry trends.
The advantages even extend to personalization. Sparrow, for instance, tailors demand forecasting to the user and product. We learned that the best synergy between user and machine happens when the machine plays a supporting role. Working as a “Co-Pilot”, it can make suggestions and recommendations that support users’ decision-making. In doing so, it infers demand for parts based on a deep understanding of the part, machine and industry—as well as the user’s own data.
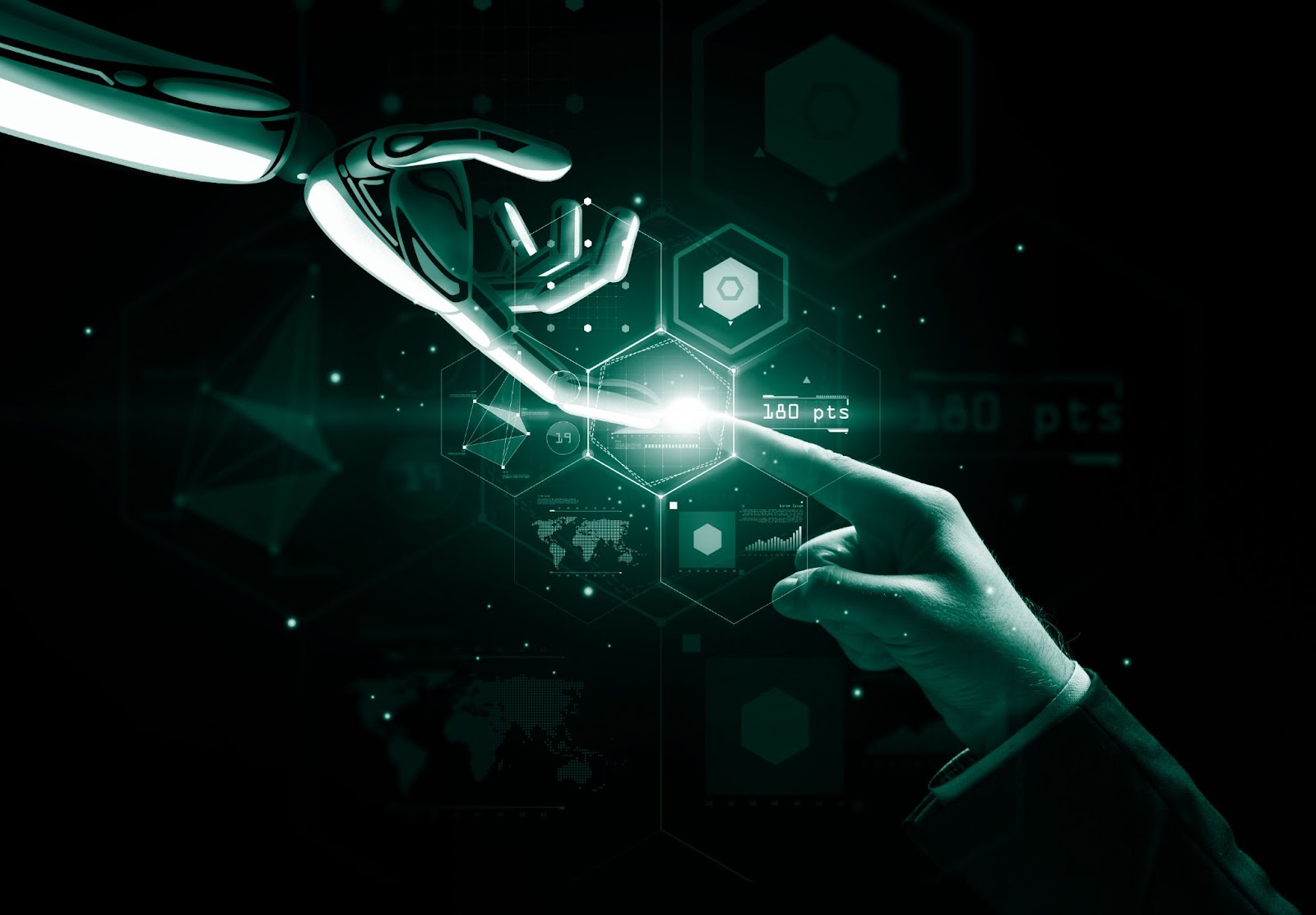
Beyond the Basics: AI’s Overlooked Advantages in Spare Parts Management
The capabilities of AI in spare parts management are not limited to inventory and decision-making. One overlooked area is logistics, where AI can centralize stocking and procurement strategies to create synergies and reduce shipping times and inventory costs. AI could eventually serve as a smart buyer that knows you well enough to automatically find the fastest and most affordable sources for your spare parts.
Challenges and Considerations of AI in Spare Parts Management
As we’ve seen, AI brings a potent cocktail of opportunities for improving spare parts management, from inventory optimization to pooling and decision-making. But we also need to to understand the potential roadblocks and limitations.
Why Data Quality Matters in Your AI Strategy
AI works with data, so it’s no surprise that the quality and timeliness of that data matter. In spare parts management, quality data leads to more accurate analytics, deeper insights, and better decision-making. Your data doesn’t have to be flawless for you to invest in it. Modern tools like Sparrow clean your data and improve it over time, leading to benefits regardless of where you stand today.
The Jigsaw Puzzle: Integration with Existing Systems
One key consideration in implementing AI in spare parts management is how seamlessly AI tools can be integrated into existing IT systems and software solutions. Lack of compatibility can lead to fragmented operations, reduced efficiency, and increased overheads in both time and financial resources. A well-planned integration process is essential to ensure that your investment in AI actually contributes to making your spare parts management more efficient.
The Human Factor: Why Your Team’s Skills Matter Just as Much as AI
Lastly, the team members who will manage and operate these AI tools need adequate training and expertise to maximize their utility. This involves not just understanding how to use the software, but also gaining insights into how AI makes decisions. Without proper training, even the most advanced AI tool can become more of a hindrance than an asset in your spare parts management operations.

What Lies Ahead: Navigating the Future of Spare Parts Management with AI
In conclusion, companies need to find ways to integrate AI in their spare parts management. Not only will this improve their processes—it could also be a competitive advantage.
Luckily, advanced solutions like Sparrow are already leveraging AI. And when it comes to increasing efficiency and responsiveness in spare parts management, these tools aren't just a fancy add-on. They're a necessity for companies looking to maintain a competitive edge in an increasingly complex industry.
Companies that incorporate AI wisely can transform traditional spare parts management challenges into catalysts for growth, efficiency, and innovation.
Interested in learning more about how Sparrow can give your spare parts management a competitive edge? Get in touch with us.